Using chatbots in the medical field - GenAI and the source verification problem
Source:
arXiv.org
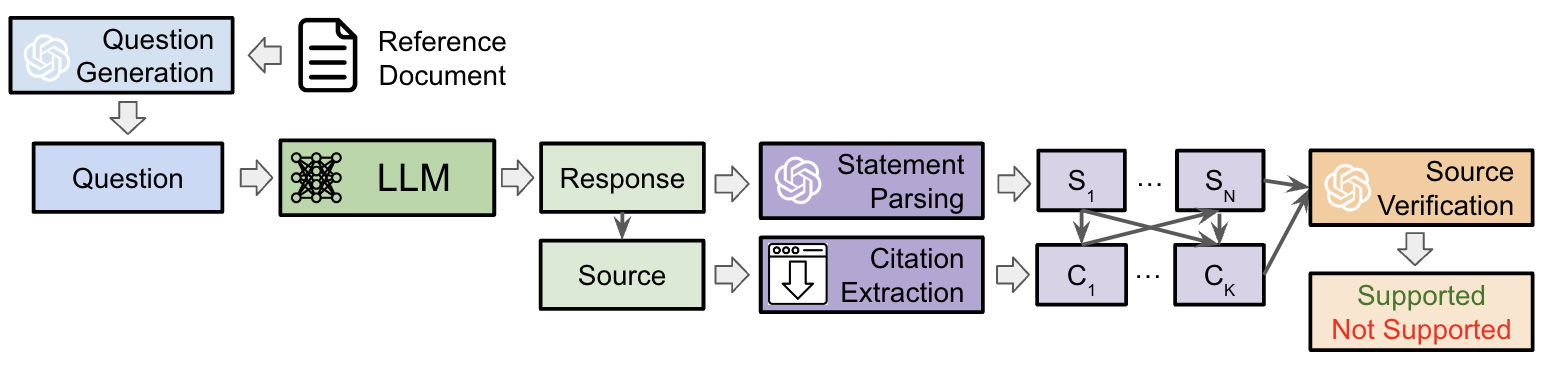
Fig.1: Diagram of the SourceCheckup evaluation pipeline.
Healthcare professionals are increasingly using chatbots to answer questions they or their patients may have. If the answers provided by generative artificial intelligence (AI) tools (GenAI) are factual and the sources cited. However, there is a risk that large language models (LLMs) may generate answers that are incorrect or lacking in veracity. This phenomenon is commonly referred to as “hallucination”.
This is the problem that researchers Kevin Wu, Eric Wu, Ally Cassasola, Angela Zhang, Kevin Wei, Teresa Nguyen, Sith Riantawan, Patricia Shi Riantawan, Daniel E. Ho, James Zou set out to address.
In this study, researchers Kevin Wu et al. set out to verify whether commercial LLMs such as ChatGPT-4 (OpenAI), Gemini Pro (Google-Alphabet), Claude v2.1 (Anthropic) or Mistral Medium (Mistral.ai) correctly indicate their results and sources.
An important point to note is that the researchers are using 2 versions of ChatGPT, one called Retrieval-Augmented Generation (RAG), which enables generative AI tools to access the Internet network in real time. Another version, known as the Application Programming Interface (API), does not offer live access to the Internet.
After recalling the operating principles of generative AI tools, researchers Kevin Wu et al. define the automated evaluation framework.
To begin, Kevin Wu et al. define an automated evaluation framework for LLMs. Named “SourceCheckup”, this automated evaluation framework consists of a set of 4 automated tasks under ChatGPT-4. This set includes:
- Question generation ;
- LLM question answering ;
- Instruction source analysis;
- Source verification.
Below are the details of the test protocol for the creation of “ SourceCheckup ”:
Module 1 - Question generation
Using ChatGPT-4 and a strict generation protocol. Document sources were framed from 3 sites:
MayoClinic, a site providing patient information ;
UpToDate, a site providing articles aimed at doctors with an in-depth level of medical detail;
Reddit r/AskDocs, a site offering spontaneous questions that often have no clearly defined answers.
Module 2 - Answering LLM questions:
Each LLM questioned must provide a short answer and an exhaustive list of their sources. A second attempt is granted when an LLM does not provide a source in its answer.
Module 3 - Analysis of instruction sources:
Decomposition of each response and analysis of each response URL in order to verify each statement. Following a very strict protocol (using ChatGPT-4), the researchers segmented each response so that they could be verified individually.
Module 4 - Source verification:
The source validation protocol comprises 3 indicators:
Indicator 1: Source URL Validity, result based on the response to an HTTP request (code 200).
Indicator 2: Statement-level support, based on the relevance of a response. For each response from a GenAI tool, the tool must provide at least 1 source of information.
Indicator 3: Response-level support, indicates the percentage of responses in which each statement is justified.
The results of Kevin Wu et al.'s research team have first of all made it possible to develop a technique for validating the results generated by LLMs using “ SourceCheckup ”.
But also, the results of the Kevin Wu et al. research team highlight the problem of hallucinations (extrapolation of results) of generative AI tools. This problem is linked to their training methods.
The final point highlighted by this scientific study is that, in the medical field, there is dissension between certain medical experts.
To begin with, GPT-4 generates a question based on a given medical reference text. Each LLM evaluated produces a response based on this question, which includes the text of the response as well as any URL sources. The LLM response is analysed for individual medical statements, while the URL sources are downloaded. Finally, the source verification model is asked to determine whether a given medical statement is supported by the source text and to give reasons for its decision.
Bibliography: (text APA)
Wu, K., Wu, E., Cassasola, A., Zhang, A., Wei, K., Nguyen, T., & Zou, J. (2024). How well do LLMs cite relevant medical references? An evaluation framework and analyses. arXiv preprint arXiv:2402.02008.
Challenges and opportunities of central bank digital currencies
Source:
Social Science Research Network - SSRN
This is a scientific study of the impact of stablecoins on central bank digital currencies. The purpose of this study is twofold, to survey the financial literature on digital assets, specifically stablecoins, and to study the interdependence of stablecoins in financial markets.
This research is authored by researchers Jaewon CHOI and Hugh Hoikwang KIM.
To back up their statements, in addition to a literary survey and analysis, the researchers have added an analysis of case studies such as the fall of Terra-Luna and Silicon Valley Bank.
Drawing on previous research, the authors begin by introducing the subject, summarizing the characteristics, advantages and disadvantages of blockchain technology.
Divided into 3 subsections, the authors address:
- The economics of blockchain technology;
- The valuation of digital assets;
- Tokenized assets and security tokens.
Concerning the literature review of the impact of stablecoins on central bank digital currencies. This part is divided into 3 subparts:
- Stablecoins and Financial Stability;
- Central Bank Digital Currency
- Prospects for Digital Assets and Stablecoins
In the 1st subpart, Stablecoins and Financial Stability, the authors focus on the technical (collateral-backed and non-collateralized) and financial characteristics of stablecoins.
The authors also discuss the impact of stablecoins on financial markets and on the decentralized finance transaction system (DeFi).
After developing the technical and financial characteristics of stablecoins, the authors tackle several subtopics in this 2nd subpart, Financial Stability. First, the advantages and disadvantages of using stablecoins as fiat currency.
Using case studies (e.g., TerraUSD - UST), the authors discuss the volatility of stablecoins, particularly algorithmic (non-collateralized) stablecoins, in the event of a simultaneous mass liquidation of stablecoins.
Secondly, the authors address the risk of the cryptocurrency market spreading to the traditional financial market.
And to conclude this subpart, the authors discuss the various regulatory systems available to avoid any financial risk on the 2 types of financial market.
In the 3rd and final subpart, Prospects for Digital Assets and Stablecoins, the authors discuss the challenges facing stablecoins and digital currencies, especially since the collapse of Silicon Valley Bank.
Bibliography: (text APA)
Jaewon CHOI and Hugh Hoikwang KIM, Stablecoins and Central Bank Digital Currency: Challenges and Opportunities (March 12, 2024). Oxford Research Encyclopedia of Economics and Finance, Forthcoming.
Available **at SSRN**: https://ssrn.com/abstract=4756822 **or** http://dx.doi.org/10.2139/ssrn.4756822
Home finance, electronic or crypto for mortage ?
Source:
Social Science Research Network - SSRN
This is a scientific study comparing traditional, electronic mortgages and those based on non-fungible token (NFT) technology.
This comparative scientific study is written by Julia Patterson Forrester Rogers, associate provost and professor of Lawat Southern Methodist University—Dedman School of Law. It should be noted that this document implies laws in force in American territory. But most of the results of the comparative study are applicable to all territories.
In this comparative scientific study, the author first highlights the advantages and disadvantages of the 3 types of mortgage loan support. Then, the author recommends solutions and updates on the laws in force in the U.S. regarding the management of promissory notes.
Divided into 5 major parts, the comparative study covers:
Part 1: The reading and comprehension problem (I. Thereading problem). In this part, the author discusses the problems of comprehension and reading, according to the different types of media, on the part of consumers. For the author, the digitization of mortgages should simplify the reading of legal clauses.
Part 2: Consisting of 3 chapters, this part covers the 3 types of mortgage loan support. In each chapter (detailed below), the author discusses the advantages and disadvantages of each type of support. The author also provides a brief history of each type of support.
Chapters in detail:
2.1 Traditional mortgage loan (II. Traditional documentation of a home mortgage loan)
2.2 Electronic mortgage (III. The transferable record)
2.3 NFT mortgage (IV. The controllable electronic record)
- Part 3: This part deals with regularization and security of the various mortgage loan vehicles (V. Control and security). After an overview of current regularizations on the various mortgage supports, the author presents the various blockchain technologies (Distributed Ledger Registration, Blockchain Registration) that fintech companies are offering. Another point covered in this section is the security of electronic and “crypto” mortgage supports.
- Part 4: The author provides a list of recommendations on the use and regulation of new mortgage loan products. The author also makes recommendations on consumer protection. (VI. Recommendations). There are 4 recommendations, the first of which is a review of the Uniform Commercial Code (UCC) regulations. Next, the U.S. Congress and the Consumer Financial Protection Bureau (CFPB) should improve consumer protection. Then, the CFPB should improve the current rules on closing residential mortgages (a problem caused by the use of electronic media). Finally, still on the subject of electronic media, the author recommends that companies managing electronic documents be better supervised by U.S. regulators.
Bibliography: (text APA)
Forrester Rogers, Julia Patterson, eMortgage and Crypto-Mortgage in Home Finance (March 29, 2024). 52 Pepperdine Law Review, Forthcoming, SMU Dedman School of Law Legal Studies Research Paper No. 644,
Available **at SSRN**: https://ssrn.com/abstract=4778343 **or** http://dx.doi.org/10.2139/ssrn.4778343
Integrating AI into business - The place of the human being
Source:
Springer Link
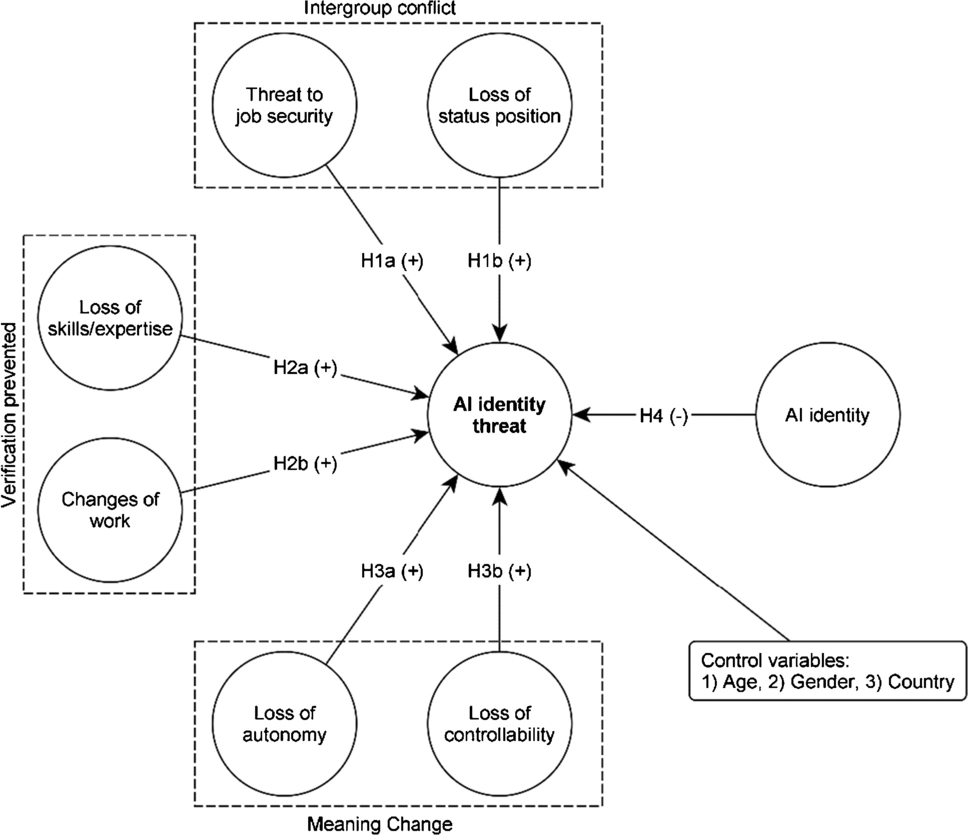
Fig.1: Research model including constructs and hypotheses as developed from the literature
The deployment of artificial intelligence (AI) in the workplace is intensifying, leading employees to collaborate more and more with AI. This interaction between machine and human augurs a new form of human-algorithm collaboration, which is not without consequences for employees.
This study by researchers Milad Mirbabaie, Felix Brünker, Nicholas R. J. Möllmann Frick and Stefan Stieglitz has 2 objectives.
The first objective is to highlight the socio-psychological impact of the use of AI-based tools on employees and their work environments.
The second objective is to enhance the scientific literature on information systems.
To validate their model, Milad Mirbabaie & al. used a data analysis method known as the PLS-SEM approach. PLS-SEM stands for Partial Least Squares - Structural Equation Models. In short, the PLS approach is based on components (predictive model) and SEM is built on covariances (confirmatory model).
After referencing the various scientific literatures addressing the socio-psychological effects of employees on the use of information technologies, as well as referencing the scientific literature on the impact of AI on human-machine interactions and on the psychological impact of employees.
Milad Mirbabaie & al. were able to study these different results using several analysis methods. To obtain their results, Milad Mirbabaie & al. used :
- Cronbach's alpha coefficient ;
- Composite reliability (or construct reliability) ;
- Rho_A (or Spearman's rank correlation coefficient).
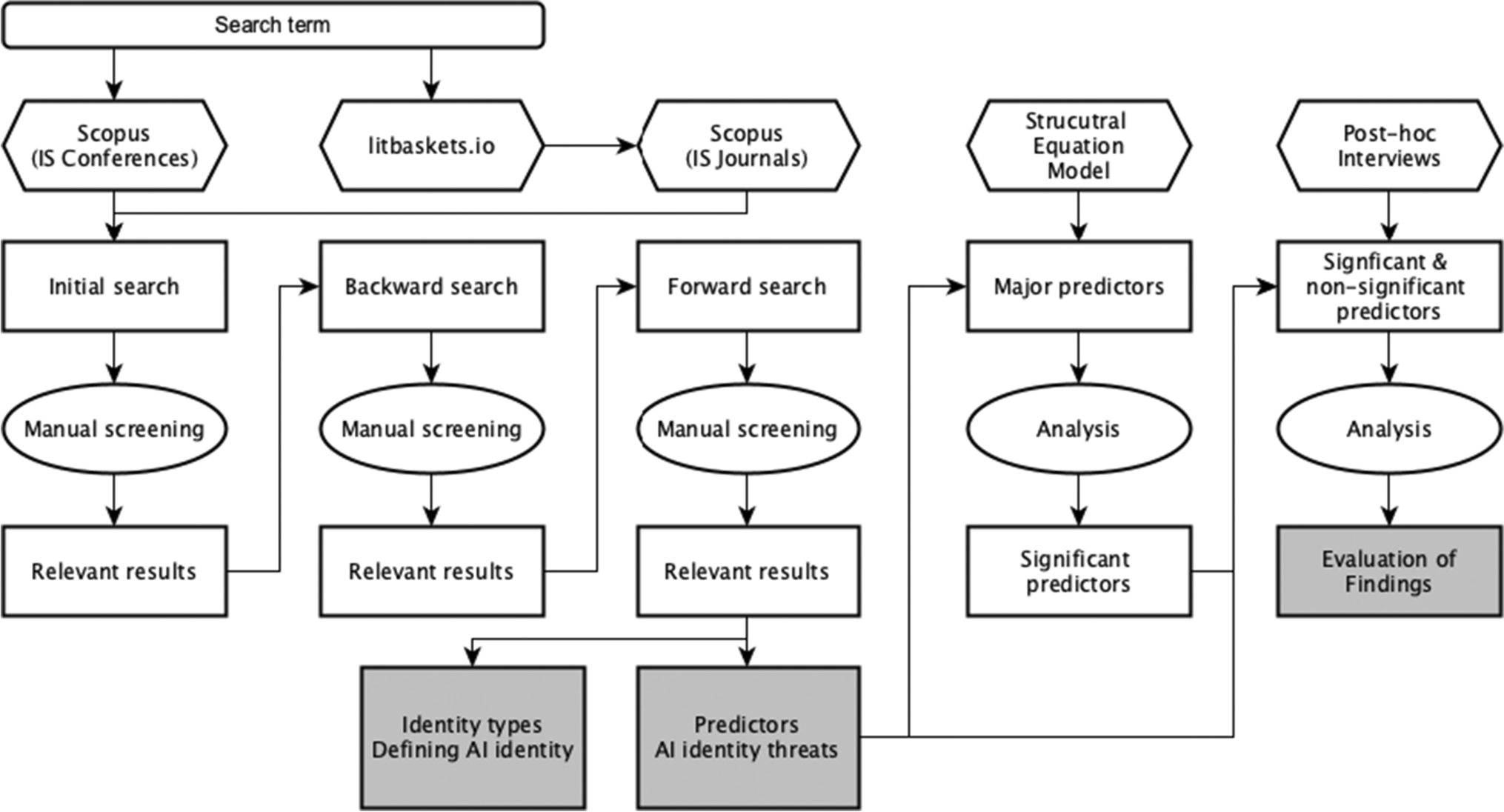
Fig.2: Procedures of the applied methods
This study also highlighted the fact that IT and AI are both distinct fields. However, it also shows that IT and AI are complementary to each other.
Bibliography: (text APA)
Mirbabaie, M., Brünker, F., Möllmann Frick, N.R.J. et al. The rise of artificial intelligence – understanding the AI identity threat at the workplace. Electron Markets 32 73–99 (2022).
https://doi.org/10.1007/s12525-021-00496-x
Generative AI tools Reducing students' creative thinking?
Source:
ScienceDirect
This scientific study was published in April 2024 in the Journal of Creativity. It is a scientific study carried out by researchers Sabrina Habib Thomas Vogel, Xiao Anli and Evelyn Thorne.
The purpose of this research is twofold. Firstly, it investigated whether the use of generative artificial intelligence (AI) tools (GenAI or Generative AI) such as OpenAI's ChatGPT or Google's BARD has any impact on human creativity, and more specifically on student creativity. Let's not forget that it's these students who will later be employed by companies.
The other aim of this research is to help teachers incorporate the use of generative AI tools into their teaching.
To carry out this study, the group of researchers selected a panel of 100 students in the first year of a course entitled “Creative Thinking & Problem Solving”.
By way of background, this course is offered at a university free of charge. The course is accessible to the whole campus, either face-to-face or in the form of a MOOC (Massive Open Online Course).
For the technical part, the researchers used the ChatGPT 3 generative AI tool. Admittedly, this is not a recent version of ChatGPT, but the operating principle remains identical to the latest versions.
As for the analysis method, the researchers used the AUT tool. Designed by J.P. Guilford in 1967, AUT is a test used to assess an individual's divergent thinking (a key element of creativity). It involves imagining the greatest number of possible uses for a common object within a given timeframe.
For example, given a “brick”, you might imagine uses such as “paperweight”, “doorstop” or “building material”.
Divergent thinking is assessed on the basis of 4 criteria:
- Fluidity: The total number of different uses you can think of.
- Originality: the degree of uniqueness or rarity of the uses.
- Flexibility: The variety of categories into which your uses fall.
- Elaboration: the amount of detail in your answers.
This test explores and measures creative potential, encouraging individuals to go beyond conventional uses and come up with innovative ideas.
The first AUT test was carried out before the students started the course, to limit the influence of teaching. Students were asked to set a timer for 3 minutes and, during this time, write down as many uses of a paper clip as they could imagine without the aid of electronics, then type in their answers to submit the work.
The same activity was repeated four weeks later, but this time they were asked to use ChatGPT-3 to help them in their brainstorming process. Students had to distinguish between their ideas and those of the AI when handing in the work, and even write a reflection on their brainstorming experience without any tools in relation to the AI's help.
The overall feedback from this scientific study was positive. Across the entire panel, there were 42 comments favorable or positive to the use of AI Generative. This compares with just 18 negative comments.
Among the positive comments, students recognized the time saved and the variety of answers provided by the use of Generative AI.
On the negative side, students highlighted the risk of plagiarism and infringement of intellectual property rights. In other words, the Generative AI-based tool is not creative. It simply provides an answer that already exists.
The use of AI in this study led to a high level of fluency (idea generation), which the students appreciated for brainstorming and idea initiation, as the AI provided a variety of detailed responses with incredible speed - less than a minute from login to responses.
However, some students expressed reservations about the AI taking over the thinking process, raising concerns that the AI might stifle their individual (human) creative thinking and, consequently, their confidence.
Although the research was aimed at the academic world, the results can also be applied to the corporate world. Indeed, tools such as OpenAI's ChatGPT or Google's BARD can also be used in brainstorming sessions or in advertising companies.
Although these results are positive, it should be borne in mind that this panel is not representative of the population. It is a homogeneous panel of students with a university degree in creativity.
On the technical side, the Generative AI tool used is not the most recent version of ChatGPT (version used: ChatGPT-3).